Problem Statement
Localising objects under fragmented occlusion is an important pre-requisite for through-foliage detection in forests. Unfortunately, state-of-the-art detectors fail to accurately localise objects under this type of occlusion.
Interestingly, human vision seems to overcome fragmented occlusion seamlessly by integrating partial visual percepts over time.
Goal
The thesis builds on previous work based on an encoder-decoder network and aims at open questions to be answered, such as:
- how to improve the method to generalise to empty scenes and scenes with more than one person,
- why does the network generalise to occluded sequences from unoccluded training data,
- how to adapt the encoder to allow visualisation of the features
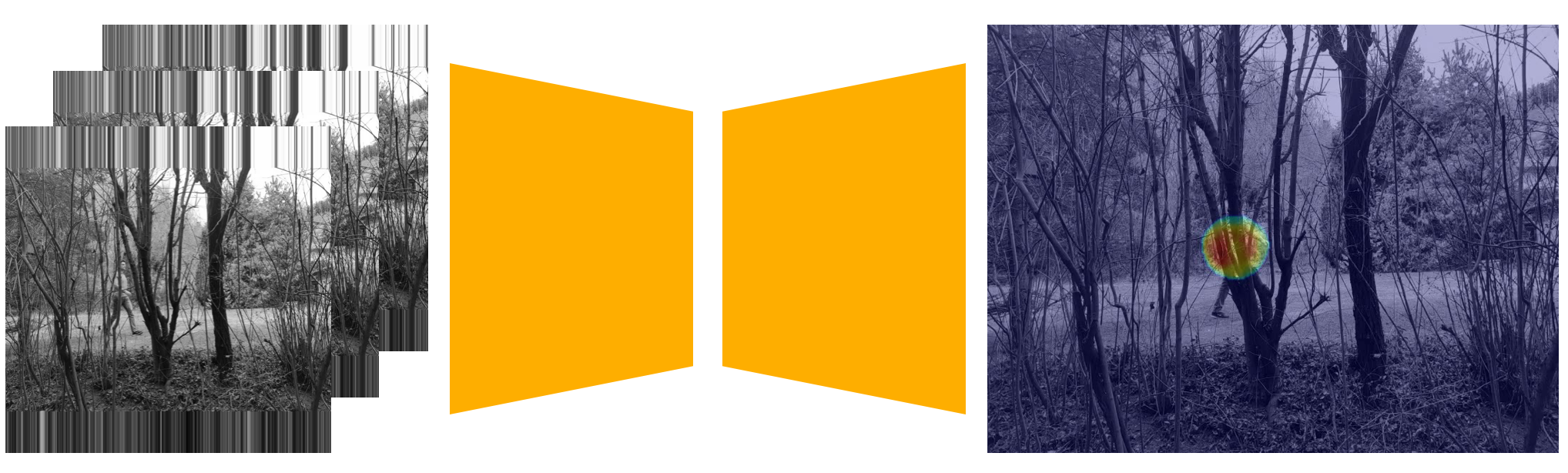
Requirements
- Basic knowledge in computer vision or computer graphics
- Basic experience in C++, Python, Julia
- Interest in machine learning, maths, statistics
Workflow
Detailed information can be found here.